The VISTA Model: A Five-Dimensional Lens for the AI-Powered Imagination Economy
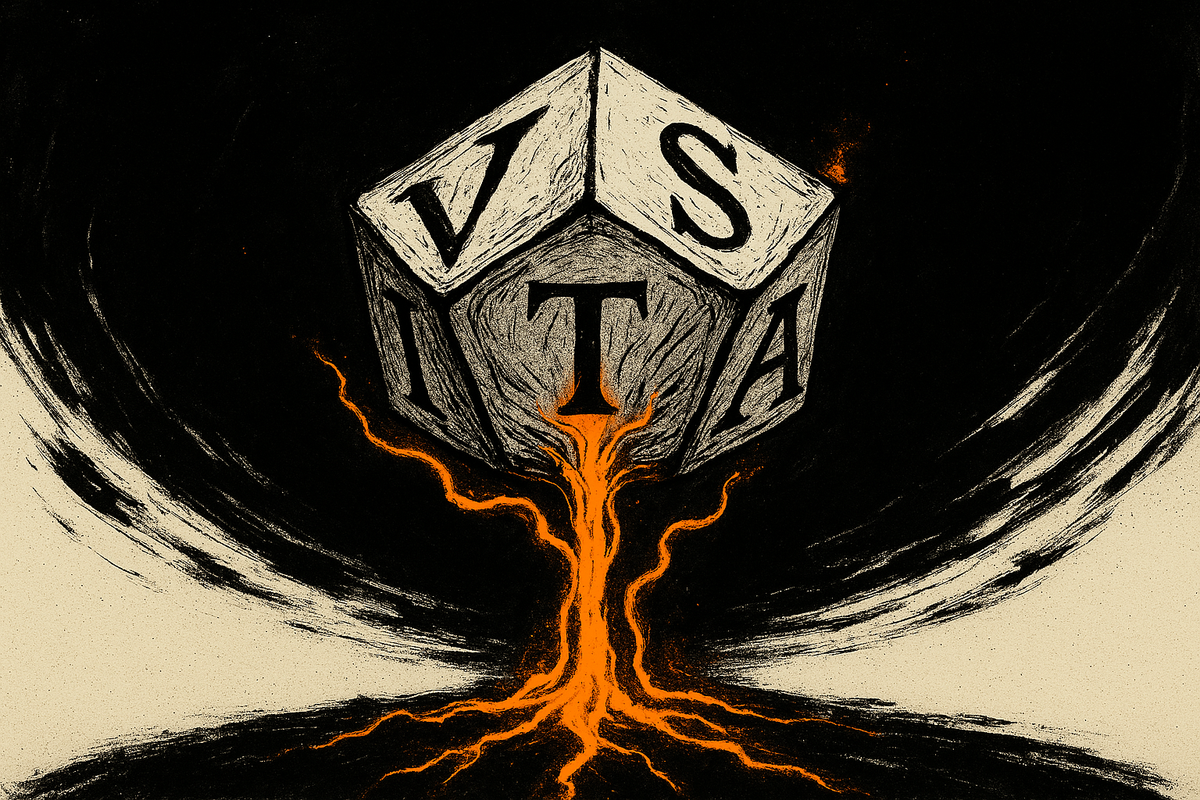
From the FLIRT Model of Crowdsourcing almost 20 years ago (long / short version), I’ve always been keen to study how technology reshapes creativity and economic value creation in society. As AI ushers in an era that I like to call the “Imagination Economy,” I wanted to take this approach to a world where ever more people can create ever more things with ever fewer constraints.
Sparring with my favorite AIs, I developed the first draft of a framework to help myself and others analyze these new kinds of innovations more rigorously. I call it VISTA, which stands for Value, Incentives, Structures, Time, and Agency, comprising a detailed approach to examine and evaluate AI initiatives and paradigms suitable for startups, enterprises, and public-sector innovations alike.
Sharing below a fairly comprehensive but still compact version of VISTA, along with reflections on how it compares to other frameworks and examples of how it can be applied across different industries. If you’ve been curious about how to structure your thinking on AI-powered creativity and economic value—and ensure no key dimension is overlooked—dive in!
Why I Created the VISTA Model
Diving into the Imagination Economy, I noticed that classic business frameworks often didn’t quite capture the interplay between new forms of creative value, shifting power dynamics, and the accelerating pace of AI innovation.
Porter’s Five Forces is excellent for analyzing competitive pressures, but it doesn’t dig into how AI changes the locus of control or how intangible experiences translate to economic results. Similarly, frameworks like PESTEL or STEEP do a great job scanning the macro environment but don’t necessarily guide you through the micro-level questions about who benefits most from AI-powered systems—or whether humans maintain meaningful agency amid AI-driven automation.
At its core, VISTA comprises five key dimensions:
- Value—the net benefit to society, companies, and/or individuals, covering both functional and experiential value.
- Incentives—how economic and psychological rewards guide stakeholder behavior and adoption.
- Structures—the institutional, regulatory, and infrastructural environment in which an innovation operates.
- Time—the dimension of when and how quickly an idea or an innovation, or different parts of it, can take hold.
- Agency—who has the power to act, decide, create, or override decisions—a critical aspect in AI-human collaboration.
The model contains much more detail, sub-questions and metrics, but below recapping some key aspects as well as real-world use cases and side-by-side comparisons against established innovation frameworks.
The VISTA Framework: Five Dimensions in Depth
1. VALUE
Key Question: What new forms of value are created, destroyed, or shifted by this innovation?
The key question is how an idea (or product) provides tangible, measurable benefit—whether that’s through cost savings or revenue upside. But unparalleled user experience and other less tangible measures need to be taken into account too. In an AI-powered world, many breakthroughs come from e.g. personalization at scale, generative creativity, and/or a productivity boost.
- Economic & Functional Value: Does this innovation reduce costs, increase efficiency, or open new revenue streams?
- Experiential & Emotional Value: Does it delight users, nurture brand loyalty, or foster a community of raving fans?
- Value Capture vs. Creation: Who in the ecosystem truly gains by capturing, not just generating value (and who might lose out).
2. INCENTIVES
Key Question: What economic, social, and psychological incentives are at play, and are they properly aligned?
No matter how brilliant an AI innovation is, it won’t gain traction if the people who need to adopt it don’t see a reason to do so. Incentives can be monetary (revenue share, profit margin, subscription savings) or intangible (reputation, creative fulfillment, community belonging).
- Stakeholder Alignment: Do customers, partners, investors, or employees gain enough to push this forward? Is there a conflict where someone loses out if the innovation succeeds? What is their power?
- Behavioral Economics: How can you create “positive friction” (e.g., encouraging reflection or critical thinking and rewarding quality over volume) or remove friction (e.g., simplifying sign-up and easing onboarding).
- Managing Externalities: In AI, negative externalities like excessive waste and energy use, misinformation or data misuse may derail even valuable concepts if not tackled proactively.
3. STRUCTURES
Key Question: Which systems, institutions, or norms enable or constrain this innovation?
Even a 10× better AI solution can be blocked if market conditions or regulatory frameworks work against it. “Structures” is where we analyze:
- Market Structure & Competition: Is this space dominated by incumbents who can squash new entrants or copy features quickly? Are there open platforms that lower entry barriers?
- Regulatory & Legal: For example, do regulations constrain user or customer data use in a given industry? Does IP law allow AI-generated content to be monetized? Are there data privacy rules that limit how you train on user data?
- Infrastructure & Organizational Culture: This includes everything from your cloud computing capacity to whether your internal org chart supports agile, cross-functional innovation.
4. TIME
Key Question: How do timing and adoption trajectories shape success or failure?
Many startups have developed a brilliant product—only to be too early in the game or miss the window when the market is already ready. They might fail to gain traction or watch competitors swoop in when the consumer sentiment catches up.
- Market Readiness: Is the enabling technology (e.g., sufficeintly advanced GPUs, devices, tech infrastructure) affordable enough and available already? Has consumer sentiment shifted?
- Diffusion Curves: Forecasts of the adoption rate and its implications. Are you expecting an S-curve over five years or a viral explosion within months? What does this mean for the business?
- First-Mover vs. Fast-Follower: Being first can yield a considerable advantage, but only if you can scale or if the product is sticky enough to deter fast-followers.
5. AGENCY
Key Question: Who has the power to act and create, and does the innovation expand or erode meaningful human agency?
This dimension might be the most unique to the VISTA framework. In an AI-driven economy, we need to ask: Who’s really in control here—humans, machines, or a handful of platform owners? And is the innovation empowering ordinary individuals or displacing them?
- Power Dynamics: Are we centralizing creativity and decision-making in a few massive AI systems, or are we distributing it through mindful structures?
- Human-Centricity: Does the tool augment people’s abilities, or does it reduce them to passive button-pushers?
- Governance & Ethics: If an AI system can make decisions on behalf of a user, how do we ensure its incentives align with the organization's goals?
Comparisons to Other Frameworks
On reflecting how VISTA differs from or complements PESTEL, Porter’s Five Forces, or Diffusion of Innovation, I see VISTA drawing on their strengths but adding key missing pieces:
- PESTEL/STEEP helps scan external factors, but it doesn’t tell you much about an innovation’s internal economics or user agency.
- Porter’s Five Forces is great for static, competition-centered analysis but less for new or emerging markets where you can change industry structure.
- Diffusion of Innovation emphasizes adoption speed but doesn’t account for incentives, power imbalances, or intangible forms of value.
At the same time, you can naturally layer VISTA onto these established tools. Do a PESTEL for the macro environment, then use VISTA to ensure you haven’t missed crucial micro-level questions around incentives or the timeline for AI adoption.
Example Cases
To make this more concrete, here are two quick examples from different industries—each showing how you might walk through VISTA’s five dimensions in each case. See if you can recognize which brands and their recent AI forays I'm talking about. Answers after the latter case.
1) A Fashion Brand Using AI Avatars of Real Models—Who Own Their Avatars
Value
- Core Offering: A global label introduces AI avatars of real models, enabling quick, customizable shoots without traditional in-person sessions. But the real models also own and control their own avatars.
- Economic/Functional Benefit: Marketers can instantly adjust shoot locations, outfits or backdrops, cutting production costs and timelines. On-set roles will shrink, but digital content creation opens up new opportunities.
- Experiential/Emotional Angle: Consumers still recognize and trust familiar faces, enriched by more varied, interactive visuals that reinforce the brand’s authenticity.
Incentives
- Fashion Brand: Gains speed and flexibility, refreshing campaigns daily if needed—potentially boosting engagement and conversions.
- Models: Owning their digital likeness ensures control over compensation and how AI avatars appear across multiple campaigns.
- Consumers: Enjoy the blend of real identity plus high-tech novelty, such as virtual try-ons with the models, that feel both personal and cutting-edge.
Structures
- Regulatory/Legal: Clear contracts must define digital likeness ownership and usage rights, setting new precedents for “digital talent.”
- Market Structure: Competitors might introduce AI avatars without model-friendly policies; a transparent, model-centric approach can be a differentiator.
- Infrastructure & Data: High-fidelity avatars require advanced 3D scanning, realistic rendering, and ample compute resources—likely involving AI studios or specialized agencies.
Time
- Market Readiness: Virtual influencers abound, but most are fictional. Bringing real models into AI form matches growing consumer acceptance of brand-led digital experiences.
- Adoption Curve: Pilot campaigns gauge consumer reaction; swift tweaks may follow if avatars feel too robotic.
- Competitive Timing: Releasing ahead of a major fashion season garners unique buzz; securing top model partnerships first can lock in exclusivity.
Agency
- Models: Control over their avatar’s appearance and usage preserves personal brand integrity, allowing them to refuse undesirable campaigns.
- Creative Teams: Still direct styling and narrative but must adapt to a digital production pipeline—upskilling in avatar creation and maintenance.
- Governance Mechanisms: Well-defined contractual terms set boundaries on edits and brand uses, ensuring genuine collaboration rather than exploitation of human identity.
2) Banking & Trading App Offering Private Banking–Level Service for the Rest of Us
- Value:
- Core Offering: An AI-driven platform that provides “private banking–style” wealth management and trading assistance—once reserved for high-net-worth individuals—to everyday users.
- Economic/Functional Benefit: By leveraging AI analytics, the app can suggest tailored portfolios, risk management strategies, and even real-time trade ideas, potentially boosting returns or reducing losses for the average retail investor.
- Experiential/Emotional Angle: Users feel a sense of financial empowerment, getting premium advice at a fraction of the usual cost. This can also build brand loyalty if the interface is user-friendly and the results are transparent.
- Incentives:
- Customers: They gain access to sophisticated tools without meeting the steep deposit requirements typical of private banking. If the AI improves returns or lowers risk, they’ll pay a subscription or small management fee.
- App Provider: Earns revenue from subscriptions, assets under management, or referral commissions. Also, a strong, trusted brand in pioneering finance can attract users.
- Financial Institutions: Some might see this as competition with their advisory arms, but others could partner to expand their retail base, especially if they lack in-house AI capabilities.
- Structures:
- Regulations: Banking and securities laws (FINRA, MiFID II, etc.) require compliance, disclaimers, and risk management frameworks. Neglecting these can shut the app down quickly.
- Market Structure: A few prominent robo-advisors exist, but many are simplistic. This more advanced, personal and refined AI approach could stand out. Large banks may replicate if the concept proves lucrative.
- Infrastructure & Data: Needs real-time market data feeds, historical pricing, and user profile data. Partnerships with data providers or brokerages are essential. Reliance on a single provider might pose a risk if that partner changes terms or cuts access.
- Time:
- Market Readiness: Post-pandemic, more retail investors became active online, and trust in AI-driven tools (like chatbots or automated assistance) has grown. This may be an ideal moment to launch.
- Adoption Curve: Early adopters might be tech-savvy, risk-taking traders who want to see how AI can care for their plain vanilla portfolios. Broader mainstream adoption will hinge on how well the AI simplifies investing for people who aren’t finance experts.
- Competition Reaction: If incumbents see considerable user traction here, they might launch their advanced AI upgrades within a year. The company must solidify user loyalty before that happens.
- Agency:
- User Empowerment: Does the app provide absolute transparency, letting users adjust risk or override trades? A black-box approach might scare people if they can’t understand what’s happening.
- Team Expertise: Domain knowledge in finance plus robust AI engineering is a must. A mismatch could lead to flawed recommendations.
- Governance Mechanisms: If the AI misreads market signals, who’s accountable? Are there built-in human or algorithmic checks (like stopping trades if volatility gets extreme)?
Answers:
1) H&M's foray into virtual models
2) Robinhood's launch of private banking
Making VISTA Actionable
Don’t just treat VISTA as a mental model; embed it into everyday decision-making:
- Build a Scorecard
- Tailor and rate the key aspects of each dimension for your environment; summarize using visual formats to visualize factors for an at-a-glance look.
- Structure Docs & Memos Around VISTA
- In decks or documents, dedicate a section to each dimension. This ensures you never skip a critical factor like user alignment or regulatory feasibility.
- Integrate Into Stage-Gate Processes
- Early in the project, emphasize Value and Incentives. Later, examine Time and Structures, and revisit Agency for stakeholder alignment.
- Onboard & Train
- Run internal or external workshops using popular cases (successful or failed). Let people see how ignoring VISTA dimensions can spell trouble.
- Iterate & Refine
- Collect real-world outcomes. If you guessed “high adoption in six months” but usage is tepid, circle back to Time or Incentives to see what's wrong.
Seeing AI Through VISTA-Colored Lenses
The Imagination Economy is more than a buzzword; it’s a shift in how creativity, AI, and human aspiration converge to form entirely new markets and cultures. With AI’s capabilities expanding so quickly, we risk overlooking hidden pitfalls—like misaligned incentives or eroded human agency—if we don’t methodically ask the right questions. That’s where VISTA can help.
When you walk through the five dimensions—Value, Incentives, Structures, Time, Agency—you get a panoramic perspective. Whether you're evaluating a scrappy fintech app that democratizes private banking, a healthcare AI for faster diagnostics, or a media platform pioneering AI-generated content, you know I’ve covered the critical angles for both feasibility and impact.
If you want to launch or invest in an AI-powered product, consider running it through VISTA. Look for big green flags (high user willingness to pay, simple but powerful incentives, excellent timing) and red flags (hostile regulations, a narrow base of domain experts, or overshadowed human agency). Each dimension can highlight strengths or weaknesses—so you can course-correct.
Thanks for reading through here!
I’d love to hear about your own experiences applying these ideas or to compare notes on how your favorite approaches might mesh with or challenge VISTA.
I can be best found at sami.viitamaki@thisisbond.com or on LinkedIn.